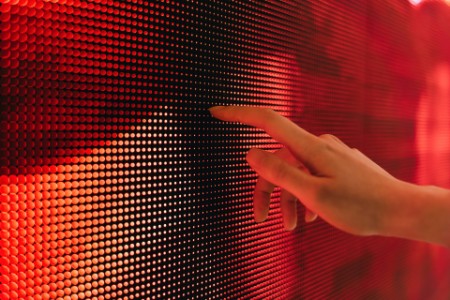
Chapter 1
The ground reality of AI in financial services
Rising AI-enabled scams underscore the need for strong regulations to address financial sector risks.
In 2023, a McAfee report revealed that 47% of Indian adults have experienced or known someone affected by AI voice scams , signaling a concerning trend. Recall the 2022 Mumbai energy company scandal, where a deepfake audio of the CEO announcing a massive price hike caused panic and chaos among investors, temporarily tanking the company’s stock. Such fabricated media have the potential to manipulate markets, erode trust, and cause financial havoc. More recently, cyber police in Kerala registered a case where scammers used AI tools in video calls, pretending to be friends, and convinced people to make online transfers.
The Organisation for Economic Cooperation and Development (OECD) AI Incident Monitor (AIM) documents AI incidents to help policymakers and all stakeholders worldwide gain valuable insights into the incidents and hazards that concretize AI risks. The following figure presents the evolution of AI incidents according to OECD analysis.
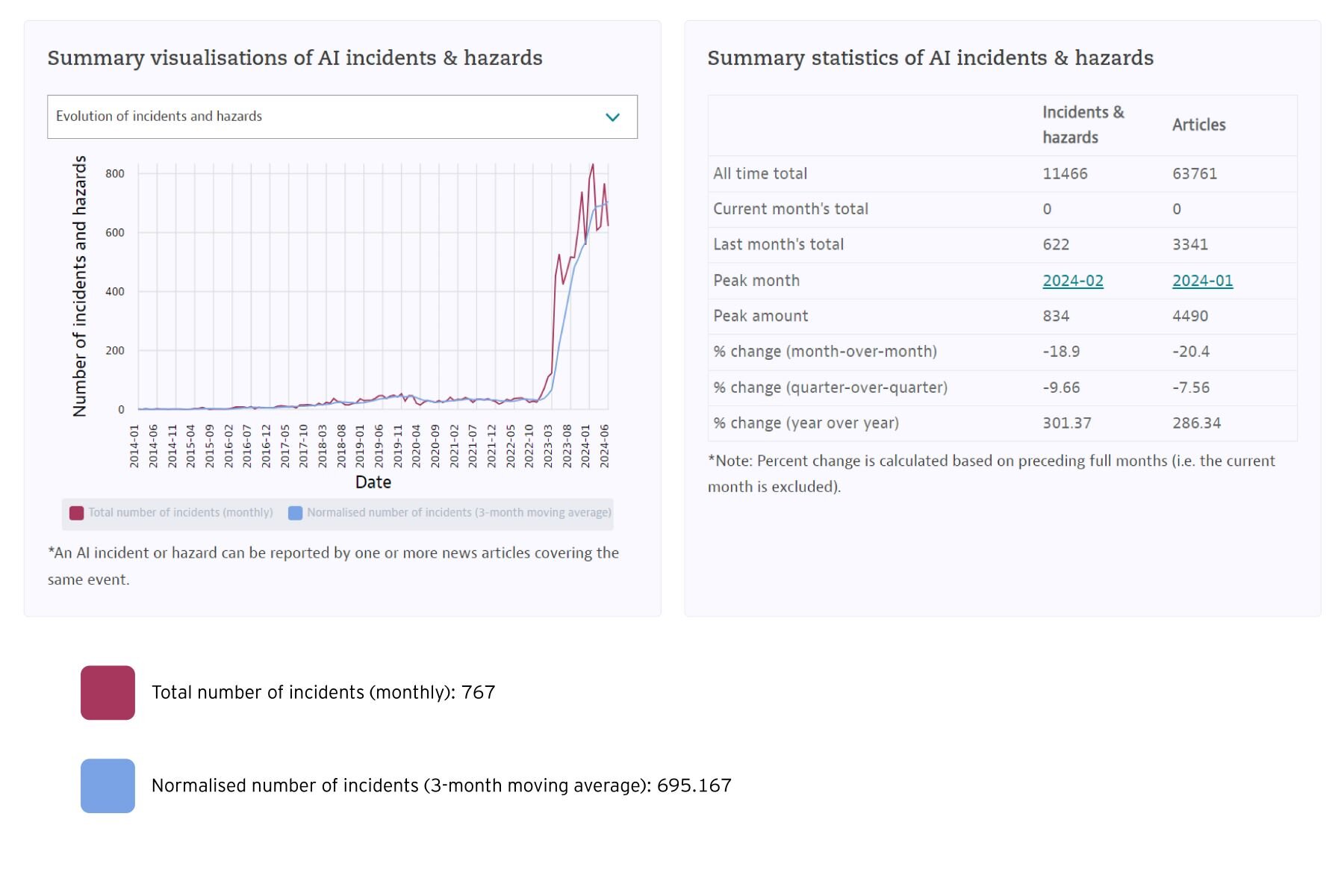
*Graph sourced from OECD.AI, a forum where countries and stakeholder groups join forces to shape trustworthy AI. Site accessed in July 2024.
From the above two panels, two things are very evident. First, there is more than an exponential increase in AI-related incidents, and second, the number of incidents in India has also risen exponentially. Considering that effective policymaking necessitates evidence-based policy formulation, it is imperative that the regulator also keep track of such incidents. However, are all risks arising from the use of AI reported or can they be captured in any monitoring system? Due to the extensive and subtle applications of AI, capturing all risks is challenging. Therefore, the regulator must also create a risk taxonomy with detailed outlines for risks, divided into different levels, and demonstrating the interconnectivity among them
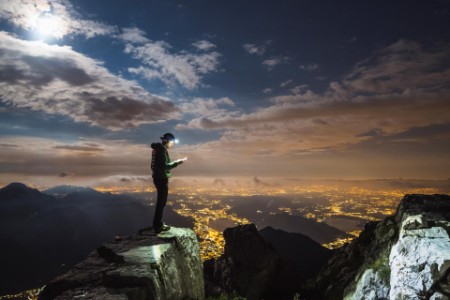
Chapter 2
Examining the global regulatory landscape
Global AI regulations focus on risk profiling, transparency, data privacy, accountability and sandboxes.
For safe and responsible AI framework, regulators across the globe have come up with several steps for risk profiling, identification and mitigation.
Risk profiling of AI use cases
The European Union's AI Act proposes a structured approach to AI risk management through a list-based categorization, dividing AI applications into four risk levels: 'unacceptable,' 'high,' 'limited,' and 'minimal.' This tiered strategy ensures that the most stringent regulatory measures are applied to high-risk AI systems, while minimal risks require less oversight. Similarly, Canada’s Artificial Intelligence and Data Act (AIDA) emphasizes accountability by classifying AI systems based on their impact, considering potential harms, the scale of deployment, and the context of use. This approach ensures that high-impact AI systems are subject to more rigorous regulatory scrutiny to protect public interests.
Fairness, explainability and transparency for AI
In terms of AI model fairness, explainability, and transparency, the G7 mandate requires AI models to undergo comprehensive fairness and bias assessments, alongside continuous monitoring to ensure ethical compliance. The National Institute of Standards and Technology (NIST) advocates for transparency and interpretability in AI systems to ensure fair and just outcomes. The General Data Protection Regulation (GDPR) strengthens this stance by granting users the right to access meaningful information about the logic involved in automated decision-making processes, promoting inclusivity and accountability.
Data, privacy and robustness of AI systems
Data privacy and robustness are critical elements in AI system design, highlighted by various global standards. NIST emphasizes the importance of designing AI systems with values such as anonymity, confidentiality, and control at their core. The Indian Digital Personal Data Protection (DPDP) Act ensures the responsible use and protection of personal data by businesses, fostering trust and security. These regulations collectively enhance the reliability and transparency of AI systems, particularly in sectors like banking, where data integrity is paramount.
Clear accountability for complex AI systems
Accountability is another cornerstone of effective AI regulation. Canada’s AIDA stresses the need for clear accountability mechanisms at every stage of AI system development and deployment. NIST emphasizes that transparency is essential for accountability, suggesting that developers must be clear about their AI applications' purposes and functions. The OECD AI Principles advocate for a clear segregation of roles and responsibilities among stakeholders, ensuring that all parties understand their duties and can be held accountable.
Regulatory sandbox
A regulatory sandbox offers a controlled environment where businesses can test innovative AI applications under the supervision of regulators. This approach allows for real-world experimentation while ensuring compliance with existing regulations. Banking regulators, for instance, can use regulatory sandboxes to oversee the deployment of AI in the banking sector, enabling banks to innovate safely. These sandboxes facilitate collaboration between the private sector and policymakers, helping to develop rules that promote safe and ethical AI use.
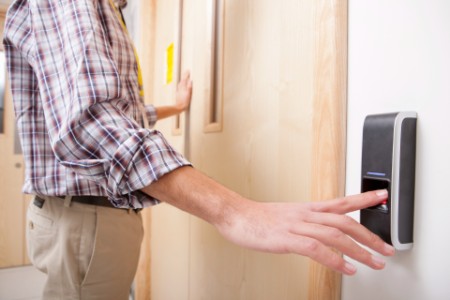
Chapter 3
Proposed regulatory framework
Regulators must enforce AI governance, risk identification, measurement and mitigation in finance.
To effectively manage the risks associated with AI in financial institutions, regulators should consider implementing a comprehensive framework based on four key pillars: governance, identification, assessment, and mitigation. This framework can be aligned with the principles outlined in the NIST AI Risk Management Framework (RMF), which emphasizes governance, mapping, measuring, and managing AI-related risks. Here is an in-depth examination of each pillar, with suggestions for regulatory guidelines:
Establish robust governance framework
Regulators should encourage financial institutions to establish robust governance frameworks to oversee AI risk management. Key areas to focus on include:
- Strong policies and procedures: Develop and enforce comprehensive policies addressing ethics, data privacy, security, and legal compliance. Ensure consistent application across the organization.
- RACI matrix creation: Implement a RACI (Responsible, Accountable, Consulted, and Informed) matrix to clearly define roles and responsibilities, enhancing accountability and decision-making in AI project management.
- Regular audits: Mandate regular internal and external audits of AI systems to assess policy compliance, identify risks, and ensure the integrity of AI models.
- Transparency and reporting: Encourage regular reporting on AI usage, risk management strategies, and audit outcomes to regulatory bodies and stakeholders, promoting transparency and accountability. By promoting these governance measures, regulators can help ensure that financial institutions develop and deploy AI systems that are ethical, transparent, and accountable, safeguarding the interests of all stakeholders.
Risk identification
Regulators should urge financial institutions to implement robust mechanisms for identifying AI-related risks. Key focus areas include:
- Unknown risks and contextual awareness: Emphasize the importance of identifying unknown risks through continuous monitoring and contextual awareness, ensuring AI systems recognize and adapt to different contexts and scenarios to operate safely and ethically. For example, a loan approval AI system should adjust its risk assessment criteria based on regional economic conditions.
- Creation of risk taxonomy: Encourage the development of a comprehensive risk taxonomy to categorize and systematically address potential AI risks, enhancing the clarity and effectiveness of risk management strategies.
- Advanced tools and techniques for risk identification: Promote the use of advanced tools and techniques specifically for identifying risks, such as:
- Machine Learning and Data Analytics: To analyze large volumes of data and detect patterns or anomalies indicative of potential risks.
- Scenario Analysis: To model different scenarios and assess potential risk exposures, identifying vulnerabilities in AI systems.
Risk measurement
Regulators should provide guidance for financial institutions on the thorough assessment of AI-related risks, linking this process to the established risk taxonomy and aligning it with the institution’s risk tolerance. Key areas to focus on include:
- Segregation of risks by responsible AI pillars: Encourage financial institutions to segregate identified risks under various pillars of responsible AI framework, such as accountability, data privacy, explainability, fairness, and robustness. This structured approach ensures comprehensive coverage of all potential risk areas.
- Prioritization and risk tiering: Suggest the prioritization of risks through a tiering system, categorizing risks into high, medium, and low tiers based on their potential impact and likelihood. This tiering helps organizations focus their resources on mitigating the most critical risks first, ensuring a strategic approach to risk management.
- Quantitative and qualitative risk measurement: Promote the use of both quantitative and qualitative techniques to measure risks within each responsible AI pillar. Quantitative methods might include statistical analysis, probability modeling and sensitivity analysis, while qualitative approaches could involve expert judgment, scenario analysis, and stakeholder consultations.
- Aggregated risk scoring: Recommend that financial institutions compute an aggregated risk score at the pillar level, based on the quantified risks. This involves integrating the results of both quantitative and qualitative assessments to derive a comprehensive risk score for each pillar.
- Overall risk assessment: Advocate for the aggregation of pillar-level risk scores to compute an overall risk score for the AI system or use case. This aggregated score should be used to inform decision-making processes, determining whether to proceed, perform further due diligence, or halt the deployment of the AI use case.
- Regular risk reviews: Advocate for periodic reviews of AI systems to reassess risks as technologies and business environments evolve. These reviews should incorporate both retrospective analysis of past performance and forward-looking assessments to anticipate future risks, ensuring that the risk measurement process remains dynamic and responsive. By focusing on these areas, regulators can ensure that financial institutions maintain a comprehensive understanding of AI-related risks, enabling them to measure, prioritize and manage these risks effectively and responsibly.
Mitigation:
Regulators should encourage financial institutions to develop and implement effective risk mitigation strategies, focusing on both pre-implementation guardrails and post-implementation monitoring. Key focus areas include:
- Pre-implementation guardrails: Advocate for embedding robust safeguards within generative AI (GenAI) platforms during the design phase. These should include bias mitigation techniques, data anonymization protocols, explainability frameworks, and adherence to ethical AI principles to prevent risks proactively.
- Post-implementation monitoring: Emphasize continuous, real-time monitoring of AI models post-deployment, including performance assessments, anomaly detection, and ongoing risk evaluation to ensure compliance and performance standards.
- Model validation and senior review forum: Recommend a comprehensive model validation framework involving rigorous back testing, stress testing, and validation against benchmarks. Establish a Senior Validation Committee, comprising executives and AI specialists, to review validation outcomes and oversee corrective actions, model recalibrations and governance measures. By focusing on these pre- and post-implementation strategies, regulators can ensure financial institutions deploy AI systems that are secure and efficient, minimizing risks and maximizing benefits through continuous oversight and rigorous validation processes.
Jatin Patni, Director of Risk Consulting, and Ravi Sundaram from Risk Consulting at EY India contributed to the article.
Related content
Summary
Regulators must take a proactive stance, encouraging financial institutions to adopt responsible AI practices. This approach fosters an environment where AI innovations can thrive securely and ethically, ultimately benefiting society as a whole.