
Chapter 1
Turning black-box models white
A look at methods to enhance explainability in AI
Machine learning models have the reputation of being a “black box” and many AI models use sophisticated techniques to arrive at answers in a way that is not transparent. As we see AI moving into all aspects of our personal and business environment, the transparency conversation is growing in importance. Decisions derived using AI models will need to be traced, challenged and immune of bias. In other words, organizations need to embrace XAI and ensure that model output can be explained appropriately to all stakeholders.
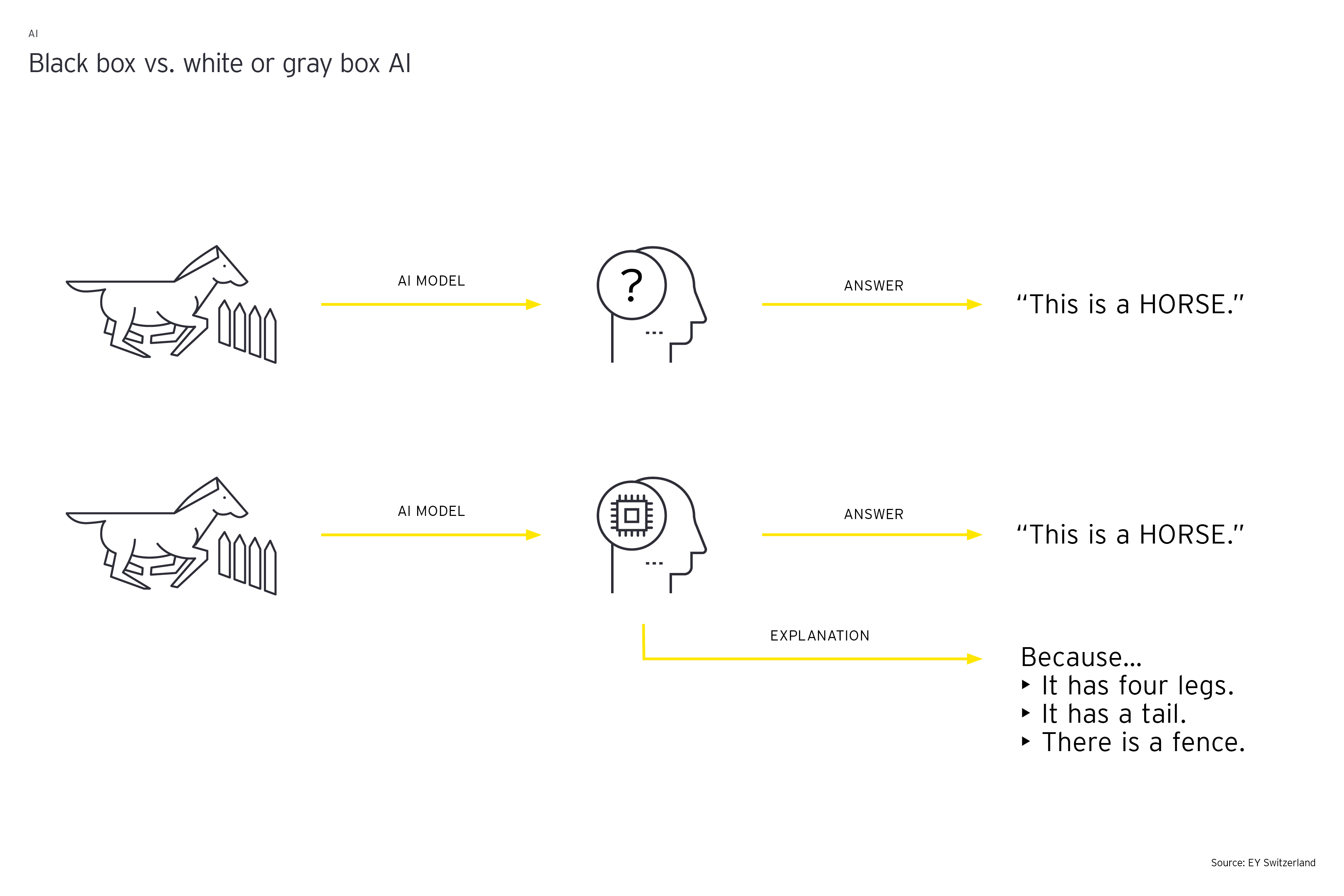
When discussing XAI, it’s important to understand the difference between:
- Global explainability
- Local explainability
Global explainability explains the overall model behavior which can help user to understand key features driving that behavior. Techniques for global explainability include feature importance and partial dependence plots.
For instance, feature importance shows which features have the highest impact on the model (but not their direction), as the chart above shows with regard to survival probability for passengers on the Titanic.
Local explainability is about a specific prediction. Techniques include local surrogate models or Shapley values. The former explains predictions of single instances by approximating the complex model by an intrinsically interpretable (– e.g. linear) – model.
Shapley values explain individual predictions by the sum of the overall average prediction and the “marginal contributions” of the individual feature values.
The above, and many other, techniques are powerful in turning a black box AI model light gray.

Chapter 2
From model design to validation
How the validation unit works
In this chapter, we discuss the AML model as an illustration of how techniques like those described above can be used.
A bank’s internal validation unit needs to understand how the AML model behaves. So, besides all other analyses, they will need to know what features drive the model’s behavior. Relevant questions in this case include:
- Are there any highly influential features that are not plausible?
- Are any features missing that a subject matter expert would expect to be relevant?
- How does the model respond when the value of features varies across their range?
- Are there any spikes in the model’s response, or is the model strictly monotonous with respect to a certain feature?
To answer the first two questions, a validator might use feature importance, while partial dependence plots can help with the latter two. These techniques enable the model to be properly challenged, which increases the chances of the model being accepted by users and regulators.
Accountability is a paramount issue in AI and we must start moving away from black-box models and toward XAI. Machine learning models are more complex than classical statistical models but there are techniques available to significantly increase transparency and transform these models into light-grey or white boxes. These techniques support the interpretation of the model behavior – useful for validation, model approval boards and regulators. At the same time, they can generate additional information that support the model users in their daily work.

Chapter 3
Spotlight on process transparency
The combined power of AI and process mining
The issue of transparency is also relevant in the day-to-day business of financial institutions, and in the digital transformation process. Before key business processes can be analyzed, transformed or automated, they must first be understood – easier said than done in today’s complex setups. Traditionally, a company’s business processes are analyzed and modeled based on interviews and workshops with key stakeholders (management, business, operations, IT etc.). While these methods provide interesting insights, they take a long time, cost a lot of money, and rarely provide the full picture of what is happening right now. There is also a strong bias in these interviews towards the official process (usually captured in Business Process Modeling (BPM) documentation) and negligence of the existing reality as the interviewees want to be seen compliant and, in many, cases designed the processes in the first place.
As most financial institutions already possess huge volumes of untapped data on their processes, it’s time to adopt a data-driven approach to understanding, analysing, transforming and managing processes. This can be achieved by combining AI with process mining. Process mining starts with an analysis of operational data that originates from event log files. Much like the records of an airplane black box, these log files capture all process steps executed on a computer system, usually in an ERP system. This data can then be used to understand process flows during process discovery. A seamless view of a process from beginning to end can be generated, allowing the financial institution to monitor the process as it operates. This way, bottlenecks, inefficiencies, errors, non-compliance and even fraud can be identified. By quickly extracting and reading these event logs, process mining software builds an instant visual model displaying the flows of events in what is known as a process graph.
AI thrives on big data and can uncover patterns hidden to the human eye, providing transparent, timely, and detailed information about the performance of processes.
In a next step, process discovery can be further enhanced. As rules-based models are heavily dependent on knowledge gained from past experiences and also have limited capability to identify “hidden” patterns, AI-based models are capable of uncovering. AI thrives on big data and can uncover patterns hidden to the human eye, providing transparent, timely, and detailed information about the performance of processes. AI algorithms applied to the discovered processes can be used as a powerful tool to identify patterns and outliers related to potential inefficiencies in speed and workload, errors, compliance issues and risks. Also, predictions algorithms using AI models applied to the as-is discovered process can then be employed to better manage resources and reduce risks.
Summary
Even though technology adoption in decision making is happening at a fast pace within financial services industry, organizations should be aware of the increasing requirement for transparency and explainability demanded by existing as well as upcoming regulations. It is crucial that organizations understand the importance and the consequences of explainable AI when considering implementing AI-based methods and models.
Many thanks to Karl Ruloff and Madhumita Jha for their valuable contribution to this article.